The future risks of AI are uncertain and hard to quantify, but that doesn’t mean policymakers shouldn’t address them now.
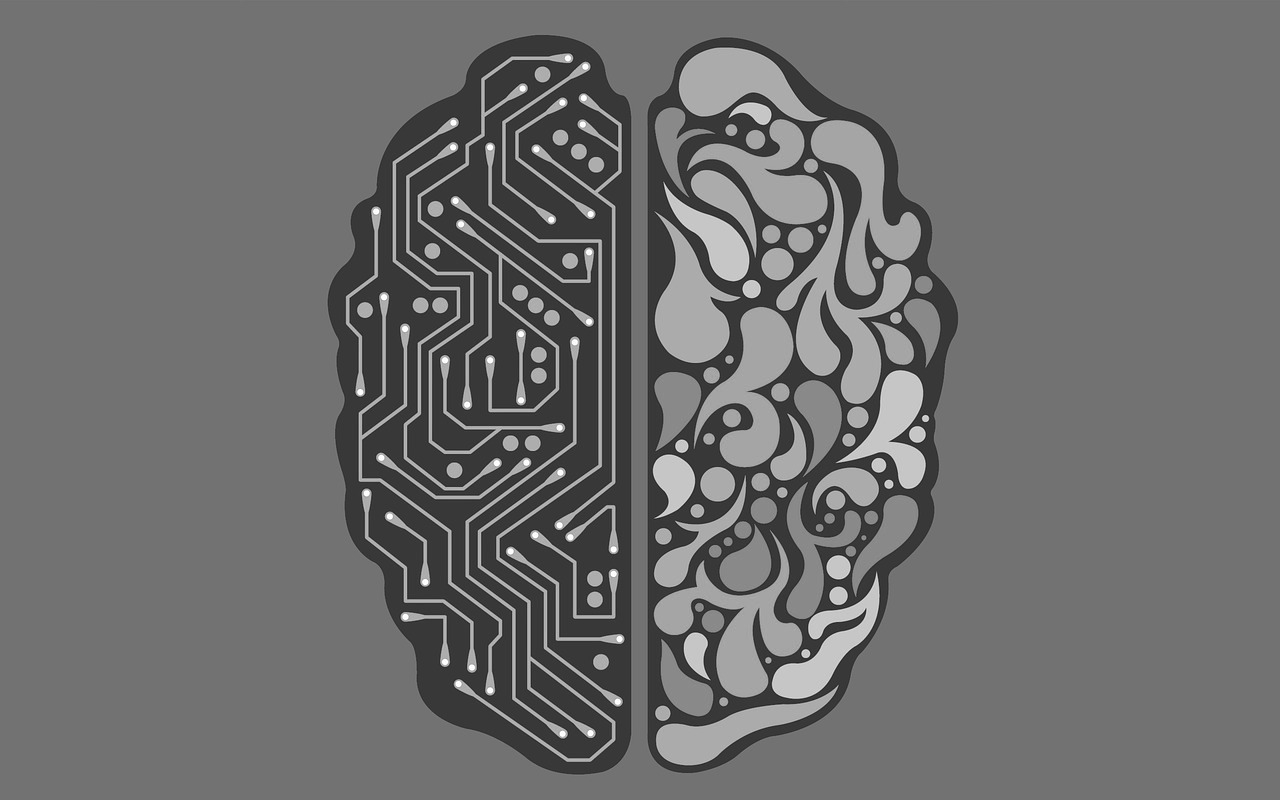
Imagine this scenario: America’s top scientists are researching a new technology on behalf of a U.S. regulatory agency. They report to the agency head that there is a substantial risk that the new technology, if deployed, could cause enormous harm to the U.S. civilian population. However, they cannot quantify the risks involved with any precision. There is a great deal of uncertainty around the potential effects of the novel technology. But the majority of researchers in the field believe that it is likely to be remarkably dangerous. Should the relevant agency, or Congress, regulate the new technology? Or, given the inherently uncertain nature of the risk, should they do nothing?
Several prominent voices in the artificial intelligence (AI) regulation debate have made versions of the latter argument, contending that policymakers should ignore catastrophic AI risks given their inherently uncertain nature. The most recent and most thorough example of this uncertainty argument was laid out a few weeks ago by the computer scientists/pundits at AI Snake Oil, and it’s worth considering in detail. Ultimately, their argument regarding the uncertainty of AI risk is flawed on its own terms, and several of its premises are mistaken, as I discuss below. But a primary issue is their failure to grapple with the history and literature of regulating risks in contexts of uncertainty. AI is not the first area where regulators or forecasters have had difficulty assigning exact numbers to the potential risks involved. And the idea of waiting until catastrophic risks are precisely quantified before addressing them is one only the most radical libertarian could approve.
Regulation Under Uncertainty
Proponents of uncertainty arguments against AI regulation generally start from a place of regulatory skepticism. For example, the AI Snake Oil pundits’ starting point in assessing regulation is that “the state should not limit people’s freedom based on controversial beliefs that reasonable people can reject.” Taken seriously, this principle would invalidate a massive swath of current laws and regulations, and prevent most new regulations. The AI Snake Oil pundits are indeed skeptical of any restrictions on AI development, although they seem to recognize a substantial obstacle to their view—that, according to a recent survey, a majority of AI experts provide a 10 percent or higher probability of humans becoming extinct or its functional equivalent in the future due to advanced AI systems. This is—to put it mildly—quite a lot of risk of a bad outcome when the bad outcome is essentially the wiping out of all human life forever.
However, the 10 percent figure is not a precise calculus of the risk involved; rather, it is an estimate in the context of substantial uncertainty. Instead of a simple mathematical output calculated via historical or experimental data, it is merely the best guess of published experts in the field. But this is no reason for policymakers to ignore it. Those who know AI technology best are all but shouting about its dangers from the rooftops. Regulators need not wait until those dangers are quantified to the last decimal place to start addressing them. Of course, regulation might be unjustified on other grounds, for example, if policymakers estimate that the (uncertain) benefits of AI innovation impeded by regulation likely outweigh AI’s (uncertain) harms. The point is simply that the existence of uncertainty surrounding a new technology or harm should not foreclose regulation.
Indeed, there is a substantial, venerable literature on regulating under conditions of uncertainty. For over a century, scholars have recognized that policymakers must sometimes act to address risks even when those risks cannot be calculated precisely. In areas such as climate change, pandemics, and genetic modification, policymakers have addressed risks that can only be estimated or guessed at rather than quantified. To be sure, the level of uncertainty surrounding AI is currently greater than that surrounding these other areas (this is discussed further in the next section). But full quantification of risk remains difficult in each context. Nonetheless, regulation is often appropriate in these contexts despite uncertainty about the future.
A widely held view within the regulatory literature is that precautionary regulatory approaches may be justified in situations of uncertainty when a new technology or practice creates a nontrivial risk of catastrophic harm. In such situations, policymakers can maximize welfare by pursuing a “maximin” strategy—that is, choosing the policy approach with the best worst-case outcome. Under this approach, if regulating a new technology with uncertain costs and benefits reduces a meaningful catastrophic risk, regulation is justified and a better option than merely waiting for the costs and benefits to become more easily quantifiable.
Artificial intelligence is a prime example of a technology for which a maximin, precautionary regulatory strategy is appropriate. The path of its future development is uncertain, and—according to a majority of experts in the AI field—it poses a substantial risk of catastrophic harm. Given these characteristics, meaningful regulation, rather than a laissez-faire approach or even a flexible, wait-and-see approach to policymaking, is currently the optimal choice. Early laws directed at catastrophic AI risk, such as California’s proposed SB 1047, which aims to impose basic safety requirements on developers of frontier AI models, are a small step in the right direction. The cautious, government-skeptical philosophy adopted by some pundits risks delaying meaningful restrictions on dangerous technologies until it is far too late.
Calculating AI Risks
While the premise of the AI Snake Oil pundits’ argument that policymakers should not regulate on the basis of uncertain risks is wrong, their specific arguments about the total unquantifiability of AI extinction risks are also flawed. Though the risks are genuinely uncertain and can only be roughly estimated, AI experts are not pulling their extinction risk estimates from thin air. They are estimating AI extinction risk the same way anyone might estimate an uncertain future risk: by reference to prior, observed events. The AI Snake Oil pundits contend that human extinction due to advanced AI is so unlike any prior event that history is no guide, at all, whatsoever. But the various components of most AI extinction scenarios—the development of supercapable AI, the failure to align that AI with human goals or safety, and the extinction of a displaced or disempowered species—are tractable, with historical precedents that are relevant and capable of providing a basis for estimation. One needs only to multiply these estimated component probabilities to produce an estimated probability of AI-caused extinction risk.
For example, someone estimating the likelihood that researchers will someday develop generally supercapable or generally superintelligent AI—defined for present purposes as AI with general capabilities or general intelligence beyond that of humans—might look to areas where current AIs or related autonomous systems already exceed human capability or intelligence in narrow domains. There are many such domains, from strategy games such as chess and Go, to many mechanical or automated tasks, to aspects of aerial and ground combat. Modern-day AIs, though sharply limited in their general capabilities, already achieve standardized test scores that exceed human averages on a vast array of cognitive and creative tests. These instances provide a relevant context from which inferences about future capability gains can be made. Likewise, previous breakthroughs in AI design, such as the development of the transformer that made modern large language models possible, provide a context for inferences regarding potential future breakthroughs. The same is true of the exponential growth of AI capabilities over the past several years. Whether such growth is predicted to continue to proceed exponentially, to slow, or to grind entirely to a halt, the fact of its existence and the history of other exponentially advancing technologies provide a basis for inferences about its future course.
Similarly, someone estimating the likelihood that a supercapable or superintelligent AI would become misaligned with human goals or interests can look to areas where existing AIs have proved to be misaligned with human goals or interests. They can also look, more broadly, at areas throughout law and society where human agents, corporations, and other entities have been unable to fully align with the goals of their principles, let alone with the interests of humanity writ large. Even confining oneself solely to the AI context, the examples are numerous and enlightening. For instance, ChatGPT seems to go “insane” from time to time, giving bizarre or nonsensical answers and insulting or threatening its users. Then there’s the AI system trained to sort data quickly that realized the quickest way to sort data was to delete it all. Or the system that learned to pretend it was inactive to avoid the scrutiny of the researchers in charge of it. My recent article (with Yonathan Arbel and Albert Lin) includes numerous other examples. In other words, there is ample relevant context for estimating the probability of AI alignment problems.
In assessing the threat to humanity from a supercapable or superintelligent AI unaligned with human interests—to the extent that this is not obvious on its face—a person might look to the history of extinctions caused by invasive species or by humanity itself to other species substantially less capable and intelligent than our own. Humans, currently the most generally capable species, affect habitats and threaten other species in countless ways. In the few hundred years since humanity came to dominate Earth’s ecosystems, the rate of extinctions of other species has increased to somewhere between 100 and 1,000 times the historical rate. Similarly, there are numerous recent examples of invasive animal species causing the extinction of native animal species that may provide a relevant backdrop for inferences regarding potential human extinction.
None of these historical instances are the same as scenarios involving AI-driven extinction, of course. They merely share some characteristics and do not share others. But the same can be said of any novel future event that experts and forecasters might try to predict. History may provide an imperfect guide for estimation, yet estimation is hardly futile. Uncertainty inevitably surrounds the future course of phenomena like climate change, or pandemics, or artificial intelligence. But when a majority of experts in these fields warn that a catastrophe may easily occur if policymakers do nothing, those policymakers should listen.
https://www.lawfaremedia.org/– Matthew Tokson, Published courtesy of Lawfare.