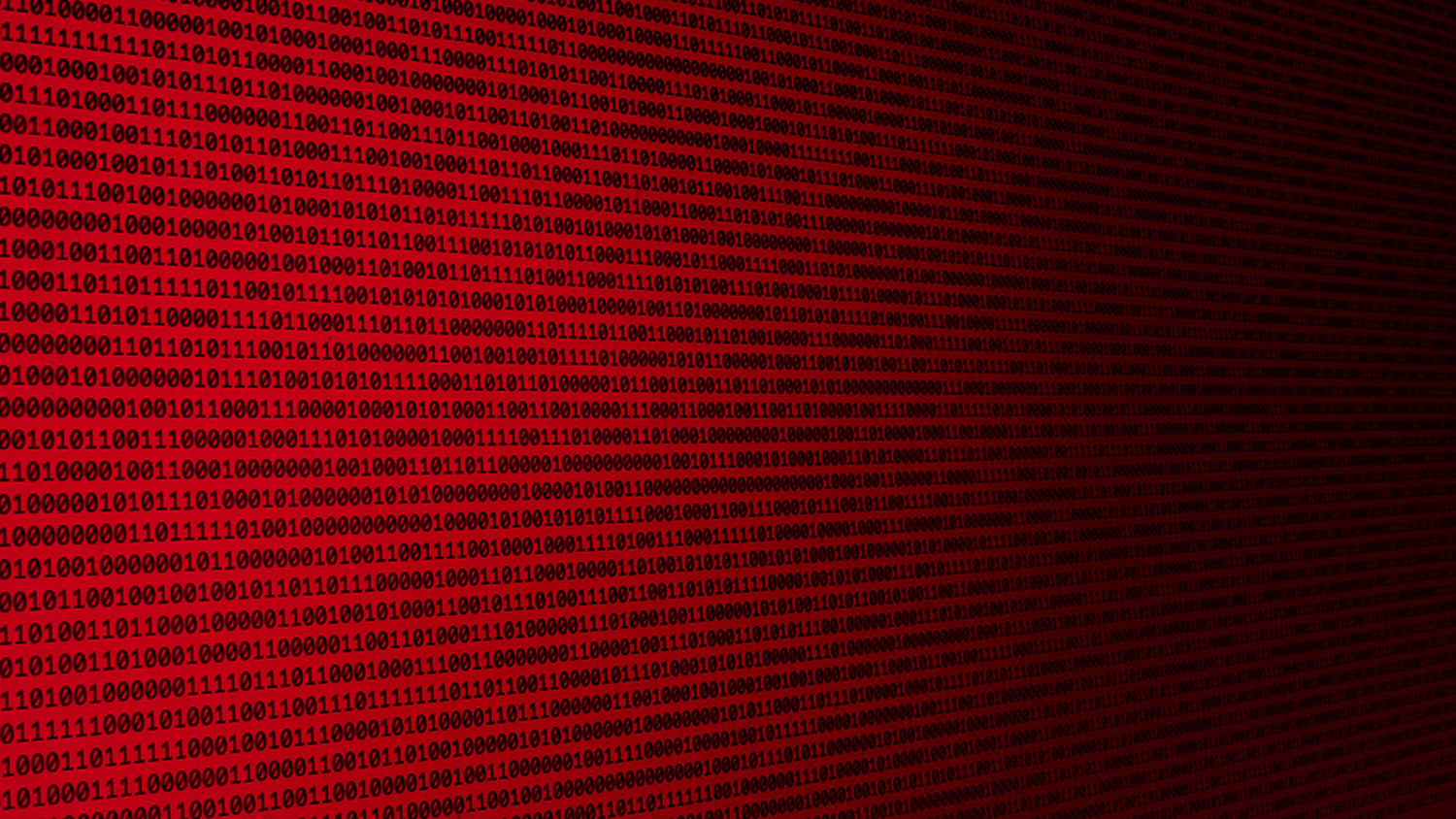
Researchers are proposing a framework that would allow users to understand the rationale behind artificial intelligence (AI) decisions. The work is significant, given the push move away from “black box” AI systems – particularly in sectors, such as military and law enforcement, where there is a need to justify decisions.
“One thing that sets our framework apart is that we make these interpretability elements part of the AI training process,” says Tianfu Wu, first author of the paper and an assistant professor of computer engineering at North Carolina State University.
“For example, under our framework, when an AI program is learning how to identify objects in images, it is also learning to localize the target object within an image, and to parse what it is about that locality that meets the target object criteria. This information is then presented alongside the result.”
In a proof-of-concept experiment, researchers incorporated the framework into the widely-used R-CNN AI object identification system. They then ran the system on two, well-established benchmark data sets.
The researchers found that incorporating the interpretability framework into the AI system did not hurt the system’s performance in terms of either time or accuracy.
“We think this is a significant step toward achieving fully transparent AI,” Wu says. “However, there are outstanding issues to address.
“For example, the framework currently has the AI show us the location of an object those aspects of the image that it considers to be distinguishing features of the target object. That’s qualitative. We’re working on ways to make this quantitative, incorporating a confidence score into the process.”
The paper, “Towards Interpretable Object Detection by Unfolding Latent Structures,” will be presented at the International Conference on Computer Vision, being held Oct. 27-Nov. 2 in Seoul, South Korea. The paper was co-authored by Xi Song, an independent researcher.