The immaturity of the AI measurement and evaluation ecosystem is a significant roadblock to the implementation of the Biden administration’s AI procurement priorities.
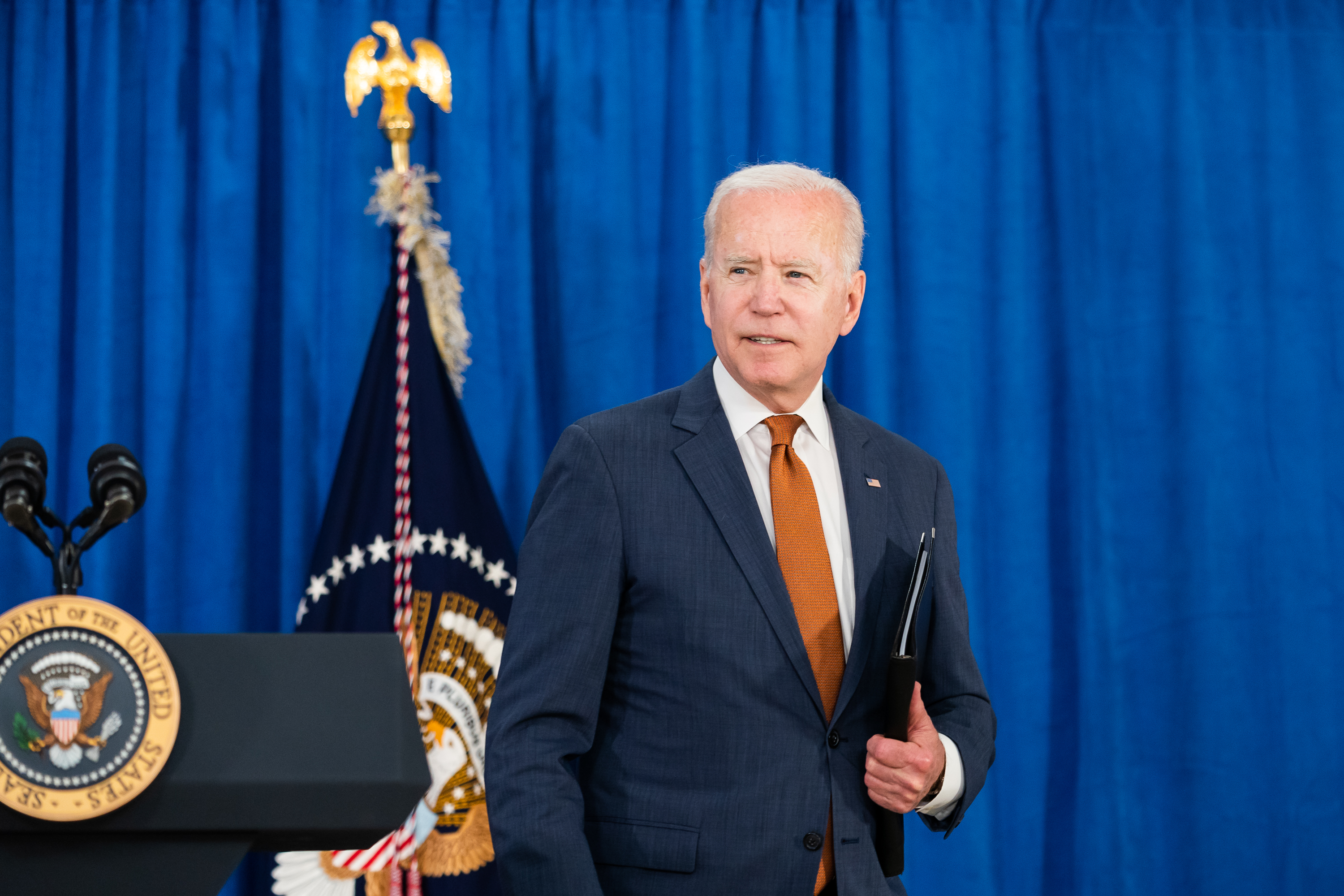
Government procurement and deployment isn’t the most headline-grabbing policy area, but these decisions carry enormous consequences. This is especially true when it comes to government use—and misuse—of artificial intelligence (AI).
A case in point: Between 2013 and 2015, one ill-conceived automated system called MiDAS wrongfully accused over 34,000 individuals of unemployment fraud in Michigan. The damage caused by this algorithmic flaw was immense: People had their credit destroyed, went bankrupt, and lost their homes. Cases like this show the importance of high ethical and safety standards matter when it comes to government AI systems.
The slew of AI-related documents released by the Biden administration over the past few years—frameworks, blueprints, guidance, a 100-plus-page executive order—makes it clear that the White House understands the significance of this problem. The need for clear rules and standards for government use of AI is a consistent theme throughout these documents, which provide a valuable starting point and high-level perspective on what to aim for.
But there’s a hitch: The science of evaluating whether a given AI system is up to scratch is still in its infancy. Tools to evaluate AI systems’ reliability, fairness, and security are currently lacking—not just within the federal government, but everywhere. Efforts like the National Institute of Standards and Technology’s (NIST’s) newly established AI Safety Institute are a step in the right direction, but the institute will need significant funding to achieve the vision laid out for it.
What Gets Measured Gets Managed
The Biden administration released its long-awaited AI executive order on Oct. 30, followed two days later by draft implementation guidance from the Office of Management and Budget. Taken together, these two documents provide some of the clearest guidance yet for agencies looking to buy and use AI. Both make clear that the federal government is interested in placing conditions on what AI systems the government will and won’t buy. But defining and enforcing those conditions could be difficult.
To understand the challenge, think of water. Everyone can agree that water should be clean and safe to drink, but stating that high-level objective is far from sufficient. To determine when water is and is not safe, we need to know three things: What factors might make it unsafe? How can we test for those factors? And what kind of test results do we consider acceptable? In the case of water, answering those questions took decades of hard work by researchers and engineers to understand the contaminants found in water, assess their effects on human health, and design devices that tell us how much lead, mercury, or microbes are in a sample. Today, the Environmental Protection Agency (EPA) keeps a long list of the things that water should not contain, each associated with detection methods and a specific concentration that is considered acceptable. The result is that—for the most part—tap water in the United States is clean and drinkable.
With AI, researchers are still in the early stages of identifying, measuring, and minimizing undesirable properties. Researchers and advocates have demonstrated a wide range of problems with AI systems, including that they can be biased, toxic, unreliable, opaque, and insecure. Many of these issues, such as ensuring that an AI system will reliably work in a new environment—for example, whether an autonomous vehicle trained and tested in fair conditions will work in a rainstorm—are still open scientific challenges despite years of research. If this were a contaminant in water, then we would know how to detect it, but not how to filter it below harmful levels. Other AI issues, like algorithmic bias, can be addressed to some extent. But these problems are often managed in an ad hoc way because standardized approaches are lacking—as if there were a burgeoning number of ways to detect and filter out different microbes from water, but no clear guidance on which microbes matter or which measurement methods are valid. Other AI challenges are foreseeable, but so nascent that we barely have tools to detect them, let alone mitigate them. The ability of AI chatbots to persuade users is one example of a property that we have almost no way to measure with current research.
From Principles to Practice
The immaturity of the AI measurement and evaluation ecosystem is a significant roadblock to the implementation of the Biden administration’s AI procurement priorities. There are plenty of high-level adjectives to go around describing what kind of AI the government should buy: The October executive order calls for “safe, secure, and trustworthy AI,” while NIST’s AI Risk Management Framework from early 2023 breaks trustworthiness down into no fewer than 11 adjectives, including “safe,” “explainable,” and “privacy-enhanced.”
These broad descriptors are a useful starting point, but they are undeniably vague. NIST’s framework does its best to help AI developers with concrete implementation, providing links to a wide range of resources that are relevant to measuring and managing risks. A “playbook” (developed alongside the framework) provides links to dozens of different ways of building or testing AI systems to be reliable, explainable, and fair. This assortment of tools is a useful repository for companies wondering where to get started in building and testing their AI systems. But without established standards on which methods to use when—and what kinds of results are acceptable—it is far from enough to allow government agencies to determine what they can and cannot buy and use. It would be as if the EPA had a water safety website linking to different brands of test kits and purifiers—better than nothing, but nowhere near sufficient.
None of this is to lay blame with NIST, which has done heroic work given extremely limited resources. The agency has been at the center of the federal government’s efforts to address the lack of high-quality, standardized measurements for AI systems, and the announcement of an AI Safety Institute within NIST shortly after the release of the executive order shows that the government is trying to rise to the challenge of developing better ways to evaluate AI systems. The new institute, announced by Secretary of Commerce Gina Raimondo at the U.K. AI Safety Summit on Nov. 1, 2023, aims to “[e]nable assessment and evaluation of test systems and prototypes to inform future AI measurement efforts.”
These are laudable goals, and NIST could be well positioned to achieve them—if it had access to funding. Notwithstanding Congress’s apparent enthusiasm for NIST as an AI hub, the agency has struggled for years with large funding shortfalls. A National Academies study completed last year described an alarming level of degradation in NIST’s buildings and infrastructure, including extensive leaks and power outages, due to insufficient funding for maintenance and upkeep over many years.
In AI, NIST’s mandate has expanded as one policy initiative after another has assigned new homework to the agency. But new funding appropriations have not kept pace, especially in the wake of October’s executive order and the announcement of the AI Safety Institute, neither of which was associated with even a penny of new funding. Senate appropriators have proposed setting aside $10 million for the institute, but even this sum—which is yet to be secured—would be a meager beginning. For comparison, the U.K. AI Safety Institute (announced at the same time as its U.S. cousin) has been promised 100 million pounds (around $125 million) per year to cover the costs of hiring scientists and engineers, running experiments on costly computational infrastructure, and building high-quality tools. If Congress is serious about making a dent in AI’s measurement problem, it will need to commit similar levels of resources. It should also look beyond NIST, for instance, by giving the National Science Foundation additional funding to support basic research that creates new ways to build and test trustworthy AI. International partners like the U.K.’s new institute can also be a valuable source of insight and potential standards.
Toward High-Quality, Multifaceted Evaluation Approaches
Of course, AI is not water. It would not make sense to try to develop a single, universal set of evaluations for a technology that can be built and used in such multifaceted ways. An AI system that processes applications for federal housing assistance should not be subject to the same tests and standards as one that recognizes wildlife from camera trap images, or one that estimates hurricane wind speeds based on satellite imagery.
But nor should evaluating AI be the Wild West. It should not be the task of individual agencies—or individual staff members within those agencies—to determine for themselves what levels of performance, reliability, disparate impact, and so on are acceptable in AI systems they are buying and developing. Stronger, more standardized tools and approaches to evaluate AI systems would go a long way to protect citizens from the risks of government use of AI.
The federal government’s push for procurement conditions aims to ensure that the AI systems the government buys are “safe, secure, and trustworthy.” But the success or failure of this push will depend on whether these high-level principles can be converted into tangible evaluation criteria. The AI Safety Institute could help reach these goals by building out the science of AI measurement, and providing the $10 million that has been proposed to fund the institute would be a good start. But this is only an early step on the path to effectively managing government use of AI.
– Matthew Burtell, Helen Toner, Published of Lawfare.