.jpeg?sfvrsn=68c7c14b_7)
Computing power—compute, for short—is a key driver of artificial intelligence (AI) progress. Over the past 13 years, the amount of compute used to train leading AI systems has increased by a factor of 350 million. This has enabled the major AI advances that have recently gained global attention. However, compute is important not only for the progress of AI but also for its governance. Governments have taken notice. As we argue in a recent paper, they are increasingly engaged in compute governance: using compute as a lever to pursue AI policy goals, such as limiting misuse risks, supporting domestic industries, or engaging in geopolitical competition.
The Biden administration introduced export controls on advanced semiconductor manufacturing equipment and the most high-end AI-relevant chips, aimed at undercutting Chinese access to leading-edge AI applications. In October 2023, the administration’s executive order “On the Safe, Secure, and Trustworthy Development and Use of Artificial Intelligence” introduced reporting requirements on models trained using more compute (1026 operations) than any that have been trained to date. Overseas, the EU’s AI Act will place additional requirements on foundation models trained using more than 1025 operations, currently covering three or four existing systems (GPT-4, Gemini Ultra, Claude 3, and Inflection 2).
States understand the importance of compute. The U.S. and the EU are both investing $50 billion in subsidies through their Chips Acts. Companies understand its importance, too. Almost all start-ups working on advanced AI have entered into “compute partnerships” with U.S. Big Tech compute providers. This includes most recently the French company Mistral, even though it had branded itself as a French-European national champion. Microsoft is reportedly investing $50 billion into expanding its Azure data centers worldwide, one of the biggest corporate infrastructure investments ever. NVIDIA has rocketed up to having the third biggest market capitalization in the world. And to boost compute production, Sam Altman is reportedly trying to raise $7 trillion.
There are at least three ways compute can be used to govern AI. Governments can:
- Track or monitor compute to gain visibility into AI development and use.
- Subsidize or limit access to compute to shape the allocation of resources across AI projects.
- Monitor activity, limit access, or build “guardrails” into hardware to enforce rules.
Compute governance is a particularly important approach to AI governance because it is feasible. Compute is detectable: Training advanced AI systems requires tens of thousands of highly advanced AI chips, which cannot be acquired or used inconspicuously. It is excludable: AI chips, being physical goods, can be given to or taken away from specific actors and in cases of specific uses. And it is quantifiable: Chips, their features, and their usage can be measured. Compute’s detectability and excludability are further enhanced by the highly concentrated structure of the AI supply chain: Very few companies are capable of producing the tools needed to design advanced chips, the machines needed to make them, or the data centers that house them.
However, just because compute can be used as a tool to govern AI doesn’t mean it should be used in all cases. Compute governance is a double-edged sword, with both potential benefits and risks: It can support widely shared goals like safety, but it can also be used to infringe on civil liberties, perpetuate existing power structures, and entrench authoritarian regimes. Indeed, some things are better ungoverned.
Compute Plays a Crucial Role in AI
Much AI progress over the past decade has resulted from significant increases in the amount of compute used to train and run AI models. Across large-language models, autonomous vehicles, and systems ranging from playing Go to predicting protein folding, the greatest breakthroughs have involved developers successfully leveraging huge amounts of computing power to train models on vast data sets to independently learn how to solve a problem, rather than hard-coding such knowledge. In many AI domains, researchers have found scaling laws: Performance on the training objective (e.g., “predict the next word”) predictably increases as the amount of compute—typically measured in the number of operations (e.g., floating point operations or FLOP) involved—used to train a model increases.
Hardware improvements and massively increased investments have resulted in the amount of compute used to train notable AI systems doubling every six months (see Figure 1). The most notable systems, ranging from AlphaGo to GPT-4, tend to be those trained using the most compute. In the past year alone, NVIDIA’s data center revenue quintupled.
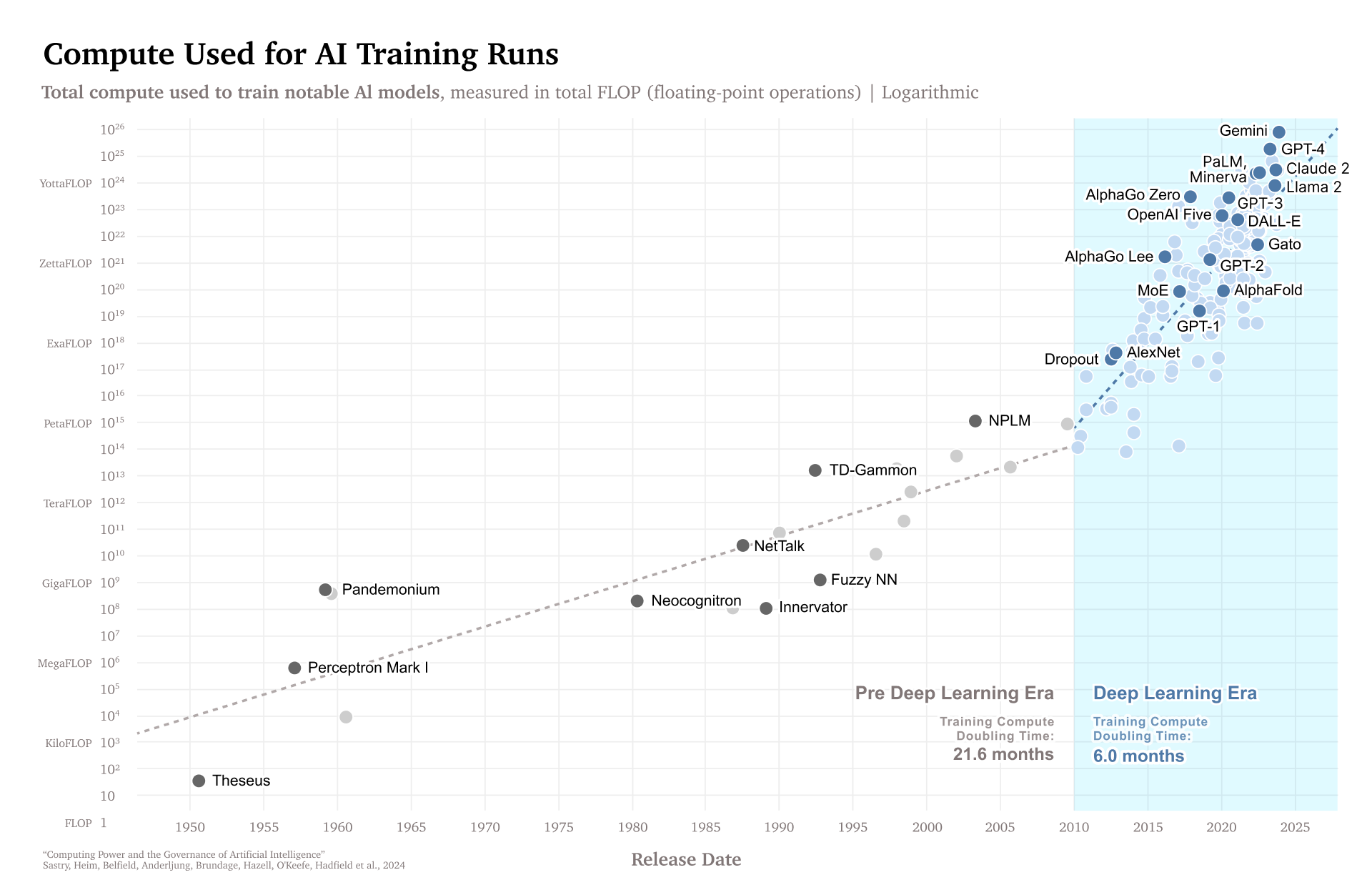
Compute Governance Is Feasible
Compute is easier to govern than other inputs to AI. As such, compute can be used as a tool for AI governance.
Four features contribute to compute’s governability:
- Detectability: Large-scale AI development is highly resource intensive and therefore detectable, often requiring thousands of specialized chips concentrated in data centers consuming large amounts of power.
- Excludability: The physical nature of hardware makes it possible to exclude users from accessing AI chips. In contrast, restricting access to data, algorithms, or models is much more difficult.
- Quantifiability: Compute can be easily measured—for example, in terms of the operations per second a chip is capable of performing or its communication bandwidth with other chips—making reporting and verification easier.
- Concentrated supply chain: AI chips are produced via a highly inelastic and complex supply chain (see Figure 2). Several key steps, including chip design, manufacturing of fabrication equipment, and chip fabrication, are dominated by a small number of actors—often just one (see Figure 3).
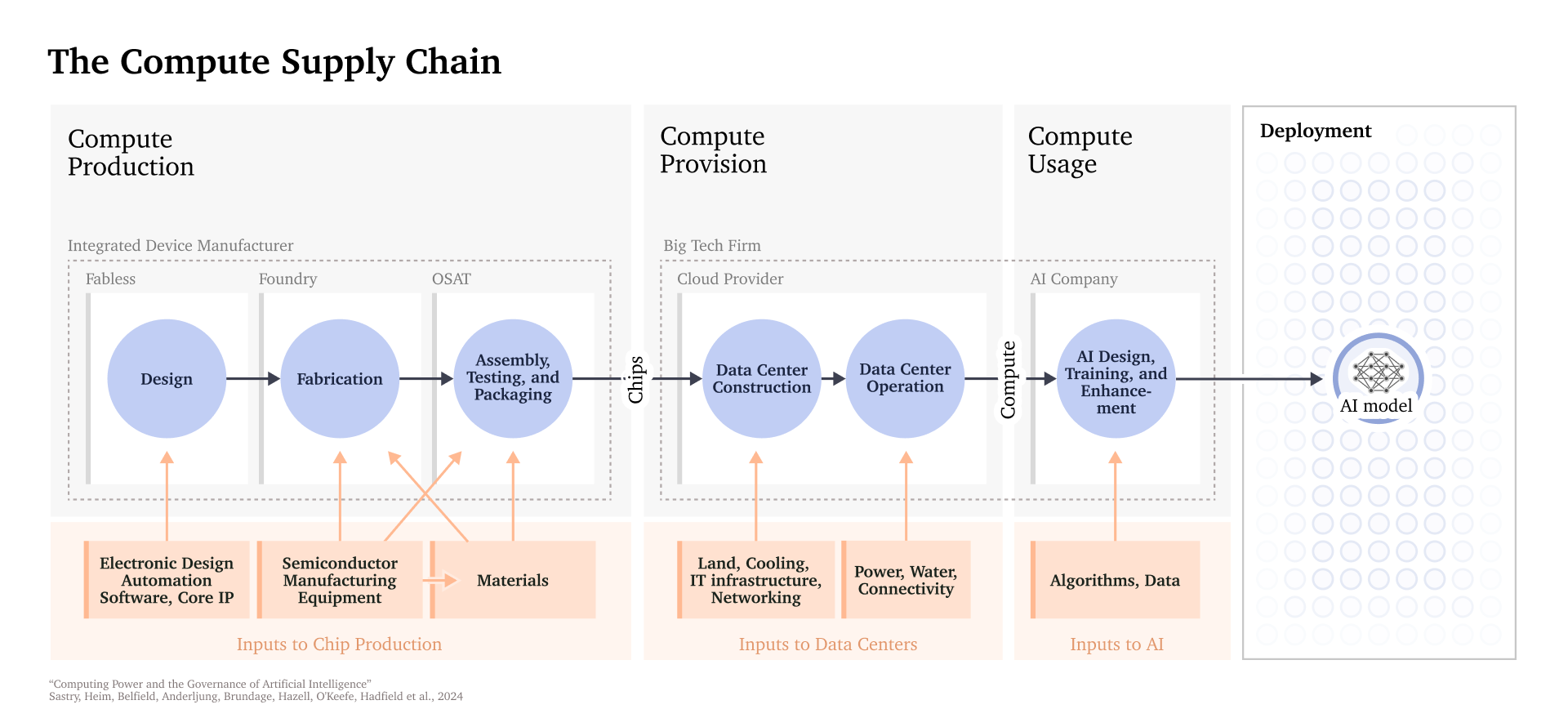
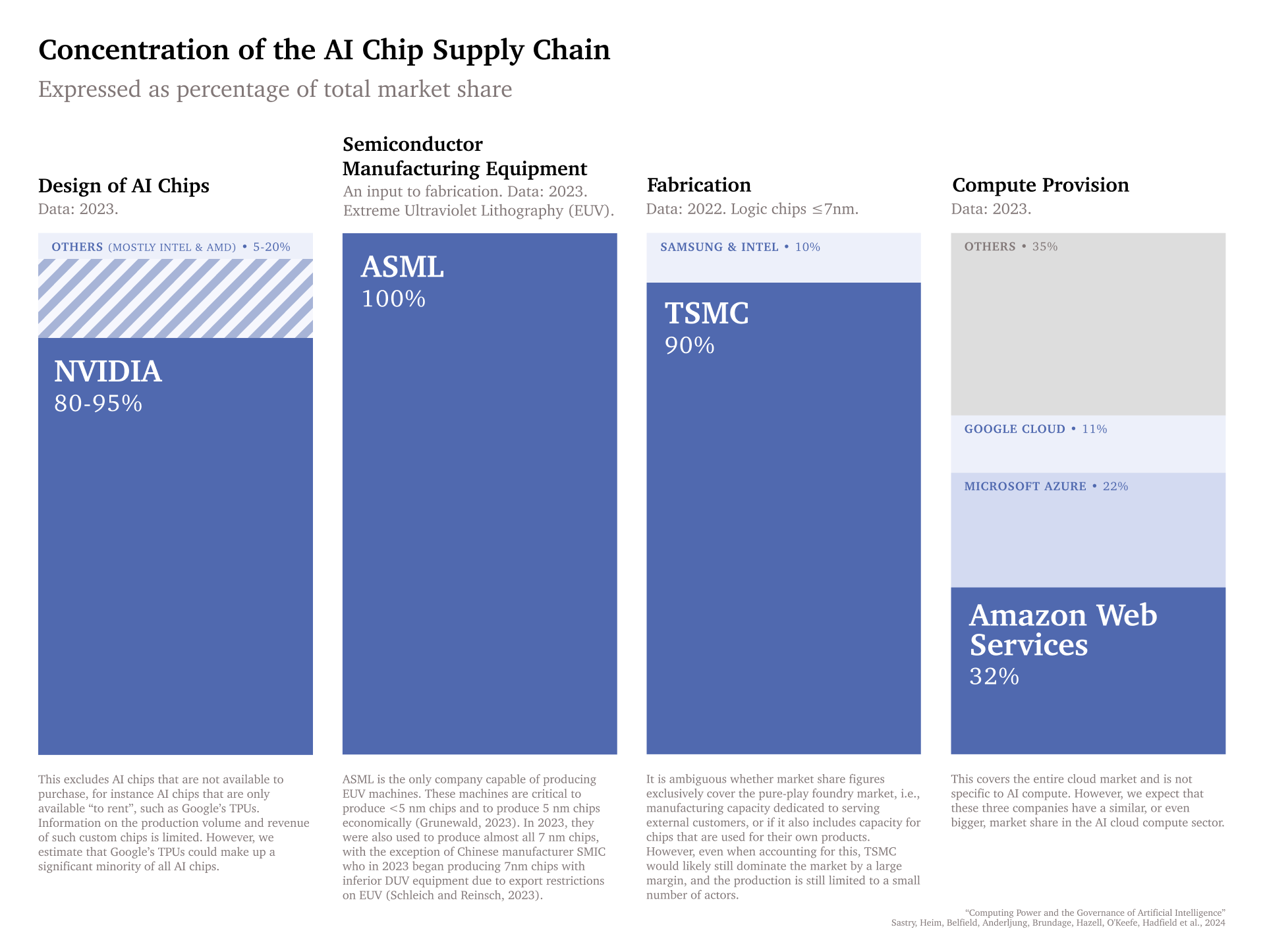
Compute Can Be Used to Achieve Many Different Governance Goals
The importance of compute to AI capabilities and the feasibility of governing it make it a key intervention point for AI governance efforts. In particular, compute governance can support three kinds of AI governance goals: It can help increase visibility into AI development and deployment, allocate AI inputs toward more desirable purposes, and enforce rules around AI development and deployment.
Visibility is the ability to understand which actors use, develop, and deploy compute-intensive AI, and how they do so. The detectability of compute allows for better visibility in several ways. For example, cloud compute providers could be required to monitor large-scale compute usage. By applying processes such as know-your-customer requirements to the cloud computing industry, governments could better identify potentially problematic or sudden advances in AI capabilities. This would, in turn, allow for faster regulatory response.
Visibility also raises important privacy concerns. Fortunately, some methods may offer noninvasive insights into compute usage. Data center operators have minimal access to information about their customers’ compute usage, such as the number and types of chips used, when those chips are used, and how much internet traffic is processed through the relevant computing cluster. However, that information can be used to glean certain insights. For example, the computational signatures of training and running inference on AI systems tend to differ. Clusters used for inference require constant internet traffic to serve customers, whereas clusters used for training typically access training data hosted locally.
Allocation is the ability to shift AI capabilities among different actors and projects to achieve some end. Once again, features of compute such as excludability and quantifiability offer promising opportunities to govern AI through allocation.
For example, policymakers may seek to differentially advance beneficial AI development by increasing the compute resources available to certain kinds of beneficial AI research and development. This could include providing access to publicly owned compute such as the U.S. National Artificial Intelligence Research Resource Pilot (NAIRR) for research into AI applications for climate change mitigation, agricultural efficiency, clean energy, public health, education, or even defense against AI misuse. Compute can also be allocated to actors lacking compute resources, such as academics, start-ups, or low- and middle-income countries.
Perhaps compute could also be allocated to adjust the pace of AI progress. A large reserve of compute could be procured by a government or an alliance of governments. The reserve could be used to modulate the amount of compute in the economy, influencing the overall pace of AI progress.
Finally, enforcement is the ability to respond to violations of norms and laws related to AI, such as reckless development and deployment or certain deliberate misuse.
One enforcement mechanism discussed in our paper is physically limiting chip-to-chip networking to make it harder to train and deploy large AI systems. For example, the U.S. government’s export controls on high-end AI-relevant chip sales to China aim to hamper Chinese actors’ ability to develop frontier AI models, where (tens of) thousands of chips are orchestrated for one training run. That goal could be met in a more targeted way by exporting chips that can only have high-bandwidth connections with a sufficiently small number of other chips. Such chips do not exist today, but could potentially be developed.
A more speculative enforcement mechanism would be preventing risky training runs via multiparty controls. Certain decisions about which AI systems to develop or deploy may be too high-stakes to leave to a single actor or individual. Instead, such decisions could be made jointly by a number of actors or a governing third party. Multisignature cryptographic protocols could be used to share control of a metaphorical “start switch” between many actors.
The power to decide how large amounts of compute are used could be allocated via digital “votes” and “vetoes,” with the aim of ensuring that the most risky training runs and inference jobs are subject to increased scrutiny. While this may appear unnecessary relative to the current state of largely unregulated AI research, there is precedent in the case of other high-risk technologies: Nuclear weapons use similar mechanisms, called permissive action links (security systems that require multiple authorized individuals in order to unlock nuclear weapons for possible use).
Compute Governance Can Be Ineffective
Although compute governance can be an effective regulatory tool, it may not always be the right one to use. It is one option among many for policymakers.
For example, compute governance may become less effective as algorithms and hardware improve. Scientific progress continually decreases the amount of computing power needed to reach any level of AI capability, as well as the cost to perform any number of computations. As the power and cost necessary to achieve any given AI capability decreases, these metrics will become less detectable and excludable.
The extent to which this effect undermines compute governance largely depends on the importance of relative versus absolute capabilities. Increases in compute efficiency make it easier and cheaper to access a certain level of capability, but as long as scaling continues to pay dividends, the highest-capability models are likely to be developed by a small number of actors, whose behavior can be governed via compute.
On a related note, compute may be an inappropriate tool to govern low-compute specialized models with dangerous capabilities. For example, AlphaFold 2 achieved superhuman performance on protein folding prediction using fewer than 1023 operations—two orders of magnitude less compute than models like GPT-4. Compute governance measures to limit the development models risk also limiting the development of similarly sized, but harmless, models. In other words, compute governance measures seem most appropriate for risks originating from a small number of compute-intensive models.
How Compute Governance Can Go Wrong
Perhaps more importantly, compute governance can also cause harm. Intrusive compute governance measures risk infringing on civil liberties, propping up the powerful, and entrenching authoritarian regimes. Indeed, some things are better ungoverned.
Certain compute governance efforts, especially those aimed at increasing visibility into AI, may increase the chance that private or sensitive personal or commercial information is leaked. AI companies, users of AI systems, and compute providers all go to great lengths to preserve the integrity of their and their customers’ data. Giving more actors access to such information raises the chance of data leakage and privacy infringement.
Large concentrations of compute are also increasingly crucial economic and political resources. Centralizing the control of this resource could pose significant risks of abuse of power by regulators, governments, and companies. Companies might engage in attempts at regulatory capture, and government officials could see increased opportunities for corrupt or power-seeking behavior.
Compute Governance Should Be Implemented With Guardrails
Fortunately, there are a number of ways to increase the chance that compute governance remains effective while reducing unintended harm. Compute governance is one tool among many available to policymakers, and it should be wielded carefully and deliberately.
Exclude small-scale AI compute and non-AI compute from governance regimes. Many of the above concerns can be addressed by applying compute governance measures in a more targeted manner; for example, by focusing on the large-scale computing resources needed to develop and deploy frontier AI systems.
Implement privacy-preserving practices and technologies. Where compute governance touches large-scale computing that contains personal information, care must be taken to minimize privacy intrusions. Take, for example, know-your-customer (KYC) regimes for cloud AI training: Applying them only to direct purchasers of large amounts of cloud AI compute capacity would impose almost no privacy burdens on consumers. KYC could also feasibly draw on indicators that are already available—such as chip hours, types of chips, and how GPUs are networked—preserving existing privacy controls for compute providers and consumers.
Focus compute-based controls where ex ante measures are justified. Compute governance (especially in its “allocation” and “enforcement” forms) is often a blunt tool and generally functions upstream of the risks it aims to mitigate and the benefits it seeks to promote. Regulatory and governance efforts typically focus on ex post mechanisms, imposing penalties after some undesirable behavior has occurred. Such measures are likely appropriate in dealing with many governance issues arising from AI, especially stemming from inappropriate use of AI systems.
However, some harms from AI may justify ex ante intervention. For example, where the harm is so large that no actor would be able to compensate for it after the fact, such as catastrophic or national security risks, ex ante measures would be appropriate.
Periodically revisit controlled compute related policies. Regulatory thresholds—such as a training compute threshold of 1026 operations—or list-based controls on technologies—such as those used in export controls—can become outdated fairly quickly as technology evolves. Compute governance efforts should therefore have built-in mechanisms for reviews and updates.
Ensure substantive and procedural safeguards. Like many government interventions, compute governance efforts could be abused. Measures to control compute should therefore include substantive and procedural safeguards. Substantively, such controls could prevent downsides of compute governance by, for example, limiting the types of controls that can be implemented, the type of information that regulators can request, and the entities subject to such regulations. Procedural safeguards could include such measures as notice and comment rulemaking, whistleblower protections, internal inspectors general and advocates for consumers within the regulator, opportunities for judicial review, advisory boards, and public reports on activities.
Conclusion
Compute governance efforts are already underway, and compute will likely continue to play a central role in the AI ecosystem, making it an increasingly important node for AI governance. Compute governance can support AI policy goals in multiple ways: by increasing visibility into AI progress, shaping the allocation of AI inputs, and enforcing rules regarding AI development and deployment. At the same time, designing effective compute governance measures is a challenging task. The risks of privacy violations and power concentration must be managed. As policymakers continue to use this tool to achieve their policy goals, they should do so with care and precision.
– Lennart Heim, Markus Anderljung, Haydn Belfield, Published of Lawfare.